Digital Twin Fundamentals-Start With Your Goal
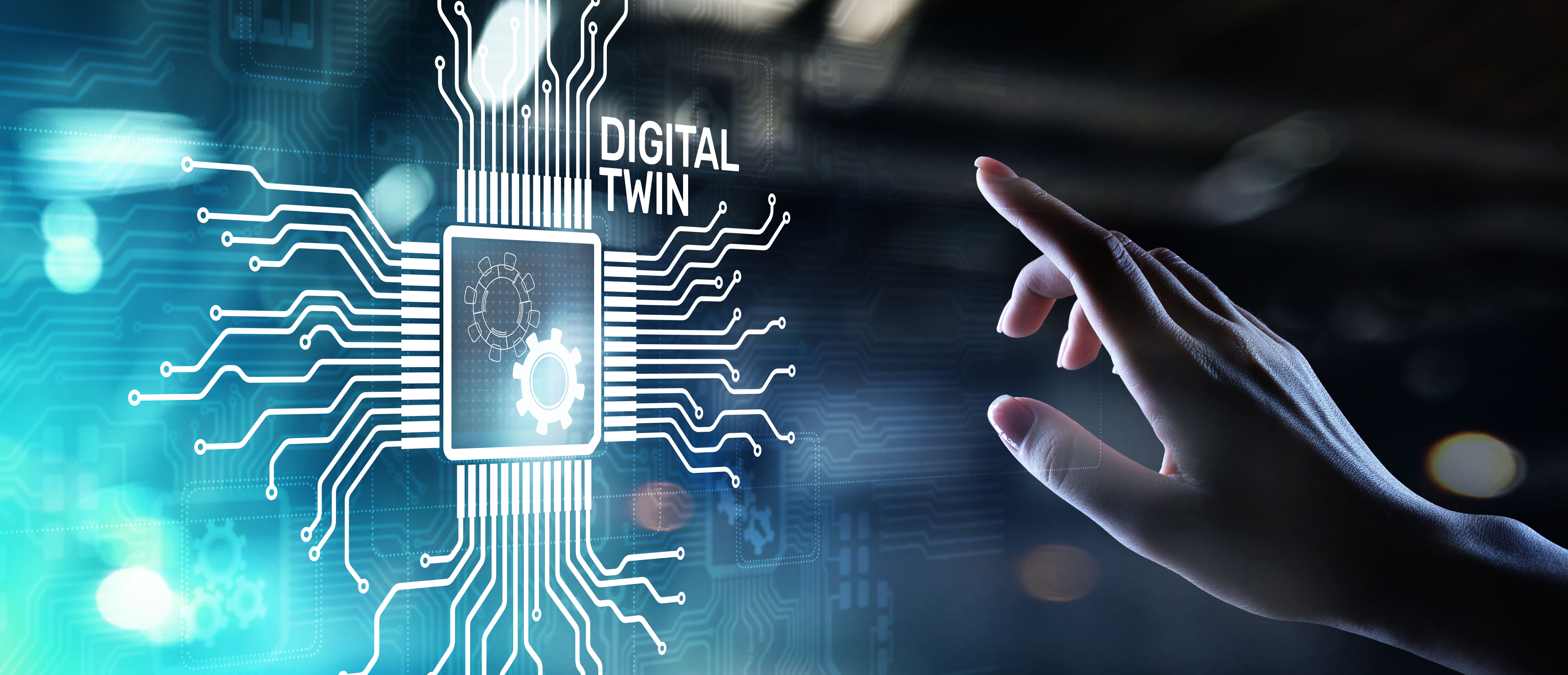
Any time you’re thinking of deploying a manufacturing intelligence platform and a full digital twin, the first step is to think of your goal.
I watched a webinar recently from the Digital Twin Consortium titled ‘Unlock Transformative Business Outcomes with Digital Twin Fundamentals’. According to the Consortium, part of the definition of a Digital Twin is ‘…digital Twins use real-time and historical data to represent the past and present and simulate predicted futures.’
Historical data in manufacturing has a clear value: traceability, compliance, and issue root-cause analysis are all driven from data that are already gathered and normalized. For predictive insights, it’s also critical to remember that building an algorithm requires extensive historical data as well. The best way to predict a future issue is to examine a related issue in the past.
For instance, if your goal is to improve yield using predictive analytics, you should examine what factors could affect yield, and how you currently measure and capture those factors. The easiest place to start is how you’ve identified and resolved yield issues in the past. If your yield has been affected by, say, bad non-serialized components, or outdated software on a tester, or by a specific firmware version on a product, what evidence in the past has told you those were the root-cause of yield issues? How did you prove that those non-serialized parts were failing early and often? How do you log and attribute that information?
You can’t improve what you can’t measure; you also can’t measure what you don’t store.