Achieving Success
With a PHased Approach
Any enterprise quality management system deployment will have lofty goals with significant impact on business processes, value, and goals. That’s why it’s critical to implement in a staged approach, achieving incremental goals based on a shared deliverables that deliver immediate business results, while future-proofing for further adoption and goal achievement..
The first step is to understand the business value of the data quality system. Using the IntraStage gap analysis team, enterprise-level customers can:
- Identify the data silos with highest ROI in quality improvement, process improvement, and yield improvement
- Interviews and data analysis to understand where the
- Identify the data silos with the easiest technical and process barriers for ETL
- Raw data requires conversion and normalization into the COTS IntraStage database and system. An easy way to achieve this conversion is by using one of our stock adapters. Manual input processes can be captured via Paperless Manufacturing
- Identify the analytics that have the highest impact in cost/labor savings
- One factor in this is how long it currently takes to produce a report. Another is the expected business value (process improvement, yield improvement) that will occur with the automated production of the report.
- Architect the data ETL process so that current and future data integrates seamlessly with other data and processes
- Understanding how individual datasets integrate with other datasets is important for understanding the full product life-cycle.
- Map out product genealogies and life cycles
- Understand which datasets are critical for go-live, and which will be implemented in further phases
- Identify data silos that could be enriched (push-pull via API) with IntraStage data or vice-versa
Using those result can develop the project plan and scorecard, where milestones and deliverables are ranked not only according to ease of implementation, but also according to the business value inherent in achieving the goals.
Technical considerations for data capture into the IntraStage database relies on a few factors: the basic format of the raw source data, the shape of the raw source data, and its relationship to other, related datasets are paramount.
deplAn example of a successful staged approach can be starting off a single set of data for adaption or with paperless forms. Another example is
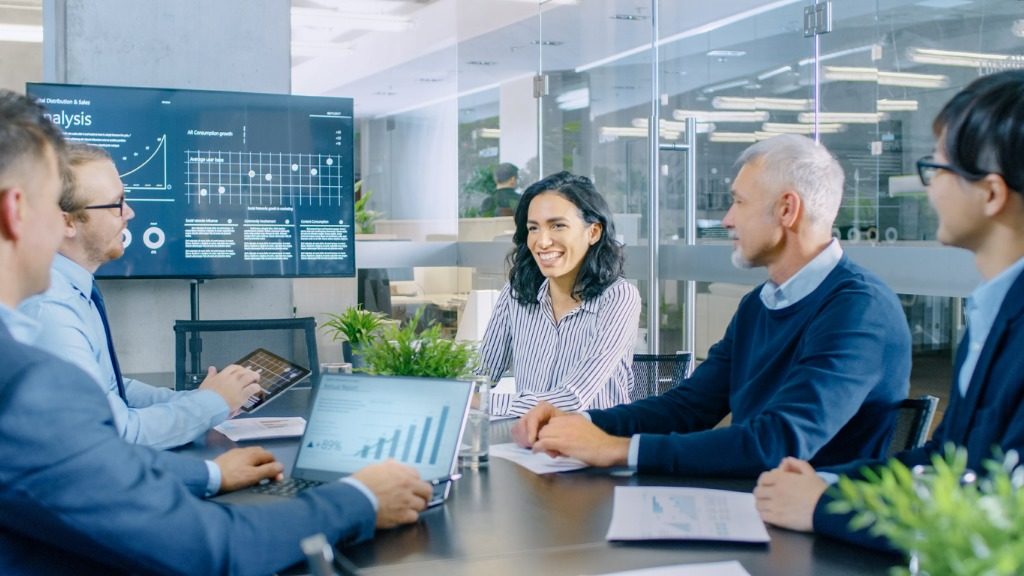