Defining the Manufacturing Data Warehouse
Defining the modern
test data warehouse
Data warehouses for electronics manufacturing are quickly becoming a focal point of IT effort and funding. And considering the potential benefits for a manufacturing enterprise, it’s not difficult to understand why. When data from multiple sources becomes necessary for critical business decisions, it’s best to aggregate and store the data in a central repository for security, reliability, and availability reasons. A data warehouse can provide:
- A centralized repository of disparate data sets, each of which can be derived from different silos.
- An architecture that provides correlation and normalization between the different data sets from the different data silos.
- On-demand deep dive analytics so the disparate data can immediately be analyzed in-depth without having to write a new query.
- Analytics agnostic access: different users can use different analytical tools (Tableau, Minitab, Excel, PowerBI, Excel, etc) to create reports and visualizations on the normalized data.
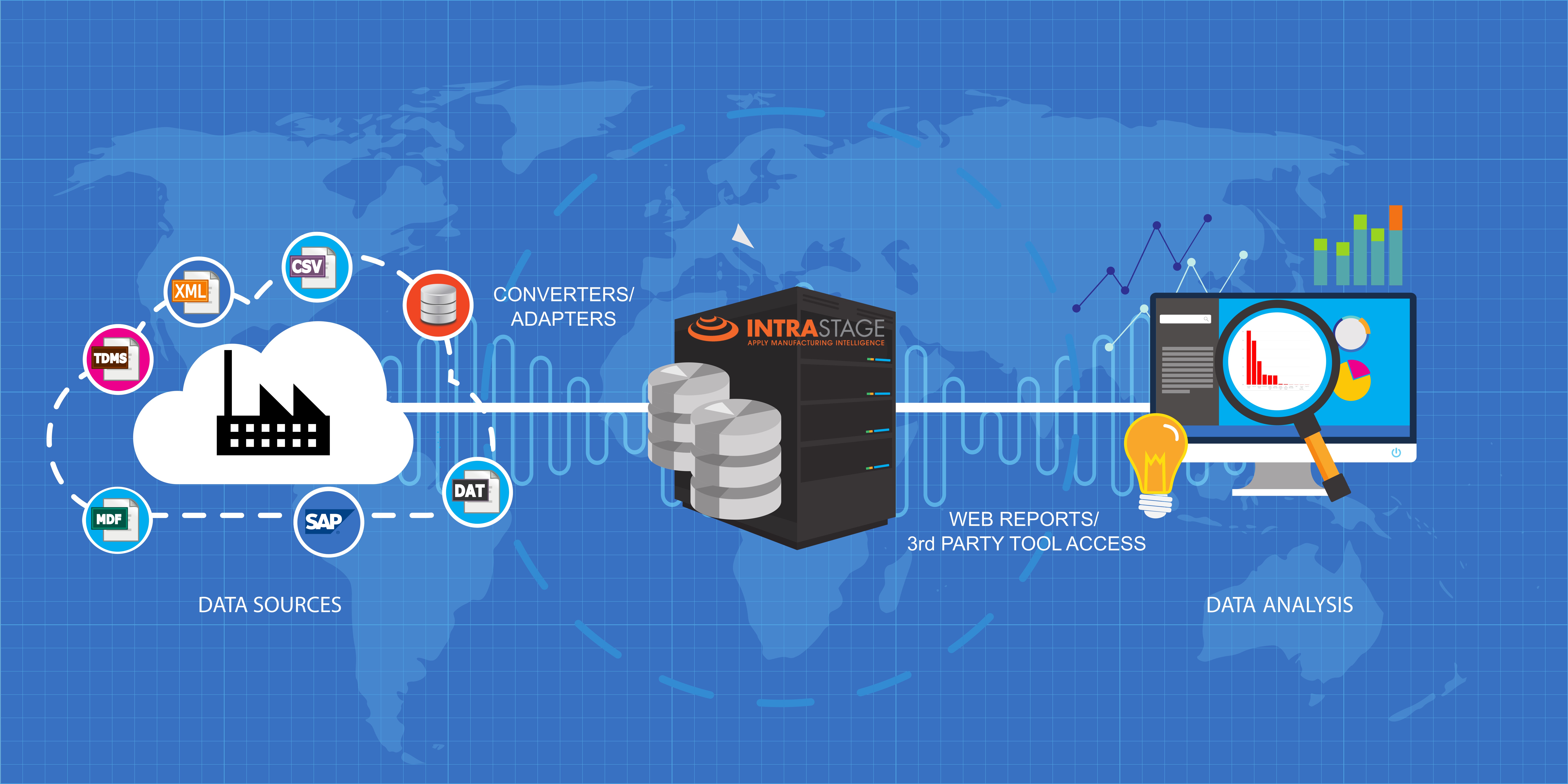
1. Centralized Data Repository
No longer is it required for a user to know where a particular data set is located and have different security credentials and have to follow different query processes to access those individual data sets. Ease of access to the data from across the enterprise should allow for quicker analysis and accomplishment of business objectives.
2 . Data Normalization
Normalizing data is critical for repeatable results and analysis, as having uncorrelated, un-normalized data will mean users would not be able to easily and quickly organize and analyze data from different datasets, and analytic results can’t easily be collaborated on, corroborated, and validated.
3. On Demand Deep Dive Analytics
When problems occur in manufacturing, time is of the essence. Every hour spent building developing queries is an hour where the production line could be stopped, resulting in massive revenue loss. It’s critical that the data be accessible, usable, and able to dive down into details of correlated data points quickly.
4. Agnostic Access
A data scientist may use complex data analysis processes and applications, but that doesn’t mean that his or her analysis will result in more value than a spreadsheet or pivot created by someone with solid domain knowledge of the source data.
About IntraStage
IntraStage is a data warehouse uniquely suited for manufacturing data. A data warehouse requires normalization, which means that the architects of the warehouse’s data model need to understand the source data and how it will be reported on and analyzed. This is not a trivial task, as it would require extensive domain knowledge of manufacturing processes and data. Rather than trying to build indexes on top of data, it’s critical to know from the beginning how the reporting and deep dive analysis will be conducted, and what the primary business cases will be. The architecture needs to account for these business cases from the lowest detail up (what am I doing with that data, and how does that data need to be structured and correlated to accomplish that analysis?) to ensure accuracy and speed. In addition, by having separate IntraStage Read tables from IntraStage Write tables, reporting and analytics can occur at the same time as data is imported, which results in better data flow and analysis without interaction between the different tables.
A high-throughput data warehouse for complex electronics manufacturers with best in class pre-built reports (and a design that is holistic in regards to other analytic tools) is available today. Contact us to learn more, and to receive a demo.